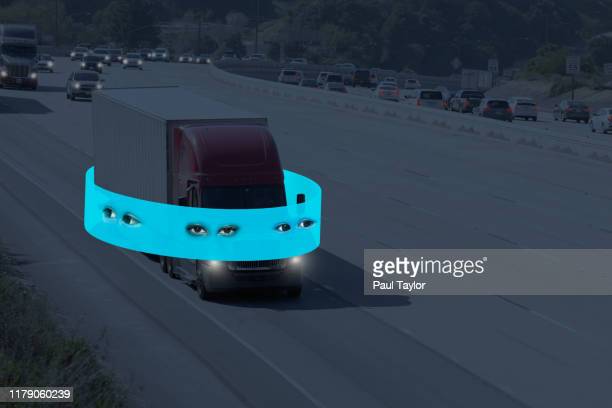
Adaptive learning is a powerful approach where AI systems dynamically adjust their behavior and learning processes based on individual needs and feedback. It enables personalized and optimized learning experiences, tailoring content, pace, and assessments to match each learner's unique requirements. By continuously adapting and refining, adaptive learning empowers learners to maximize their potential and achieve better outcomes.
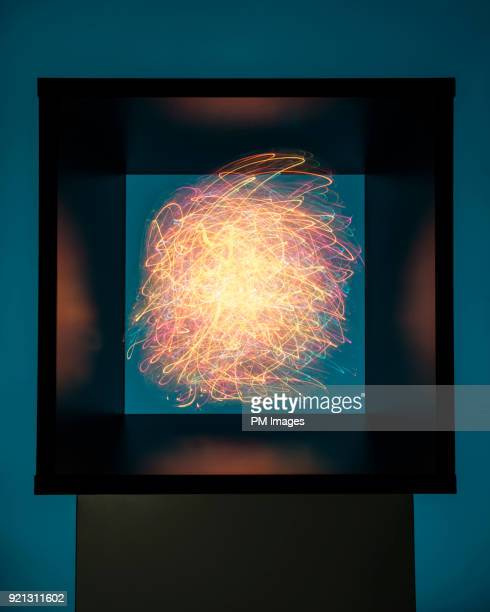
The Black Box in AI refers to the lack of transparency and interpretability in intelligent systems. AI algorithms learn from data, making it difficult to trace decision-making. This poses implications for critical applications and raises ethical concerns. Approaches like Explainable AI, model transparency, regulatory frameworks, and open-source initiatives aim to address the Black Box problem. These efforts provide human-understandable explanations, improve model interpretability, ensure compliance, and foster transparency. Unveiling the secrets within the Black Box is vital for building trust and deploying AI responsibly.
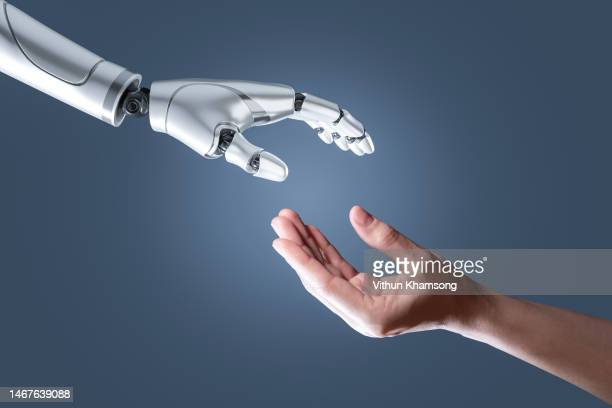
Explainable AI (XAI) is a field of research that seeks to make AI models more transparent and interpretable. By improving the explainability of AI, XAI aims to enhance trust, accountability, and ethical considerations in AI development and deployment. XAI techniques can be applied to a wide range of AI models and applications, including natural language processing, image recognition, and predictive modeling.
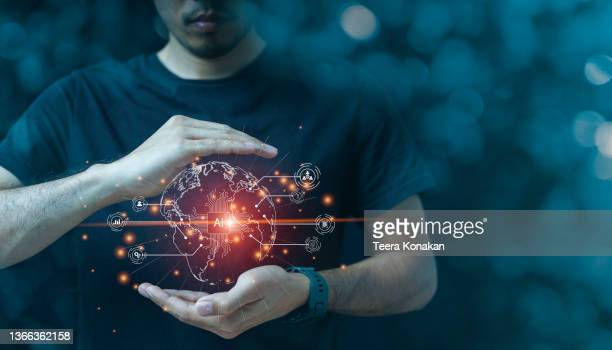
Building trust in artificial intelligence (AI) is crucial for its widespread adoption, particularly in sensitive industries such as healthcare and finance. One potential solution to this problem is to use blockchain technology to create a transparent and immutable record of an AI system's decision-making process.