Quote:
“The development of full artificial intelligence could spell the end of the human race. It would take off on its own, and re-design itself at an ever-increasing rate. Humans, who are limited by slow biological evolution, couldn’t compete and would be superseded.” – Stephen Hawking
This quote highlights the potential risks and critical issues associated with the development of advanced AI systems, including the potential for AI to become uncontrollable and outpace human intelligence. It underscores the importance of considering the ethical and societal implications of AI and taking steps to ensure that it is developed and used in a responsible and safe manner.
Table of Contents
1. Introduction
A. Overview of AI and its growing importance in society
Artificial intelligence (AI) is a rapidly advancing field of computer science that aims to create intelligent machines that can perform tasks that typically require human intelligence, such as visual perception, speech recognition, decision-making, and language translation. AI is increasingly being used in a wide range of applications, from personal assistants and smart homes to healthcare, finance, and transportation.
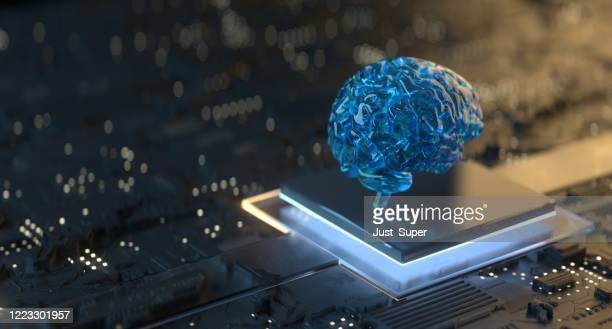
B. Brief overview of critical issues related to AI
Despite its potential benefits, AI also raises a number of critical issues and challenges. These include ethical and social implications, such as bias and discrimination in AI algorithms, privacy concerns, and the impact of AI on employment and the economy. Legal and regulatory challenges include issues related to intellectual property, liability and responsibility for AI decisions, and the regulation of autonomous systems and AI applications. Technical challenges include the reliability and interpretability of AI systems, the robustness and security of AI systems, and the need for explainability and transparency in AI decision-making.
C. Purpose and scope of the blog post
The purpose of this blog post is to explore the critical issues related to AI and provide insights and recommendations for navigating these issues in a responsible and ethical manner. The post will cover a range of topics, including ethical and social implications, legal and regulatory challenges, and technical challenges. Case studies and examples will be used to illustrate real-world applications of AI and the impact on critical issues. The post will also provide recommendations for policymakers, researchers, and industry leaders to promote responsible and ethical development and use of AI.
2. Ethical and Social Implications of AI
A. Bias and discrimination in AI algorithms
One of the major ethical and social implications of AI is the potential for bias and discrimination in AI algorithms. AI systems are only as good as the data they are trained on, and if the data used to train an AI system is biased, the system will also be biased. This can result in discriminatory outcomes in areas such as employment, housing, and lending. It is important to address bias and discrimination in AI algorithms to ensure that AI is used in a fair and equitable manner.
B. Privacy concerns and data protection
Another ethical and social implication of AI is the potential for privacy violations and data breaches. AI systems require large amounts of data to be effective, and this data can include personal and sensitive information. There is a risk that this data can be misused or stolen, which can have serious consequences for individuals and society as a whole. It is important to have robust data protection policies and regulations in place to ensure that personal data is collected, stored, and used in a responsible and ethical manner.
C. Impact of AI on employment and the economy
AI also has the potential to significantly impact employment and the economy. While AI has the potential to create new jobs and industries, it can also lead to job displacement as tasks that were previously performed by humans are automated. This can have a significant impact on individuals and communities that rely on certain industries or types of work. It is important to consider the potential impacts of AI on employment and the economy and develop strategies to mitigate any negative effects.
D. Social and cultural impacts of AI
Finally, AI also has the potential to impact society and culture in significant ways. For example, AI systems that mimic human communication can have an impact on how we interact with each other and can blur the line between what is real and what is artificial. AI systems can also be used for propaganda or disinformation campaigns, which can have serious consequences for democracy and social cohesion. It is important to consider the social and cultural impacts of AI and to develop ethical guidelines and regulations that promote responsible and ethical use of AI.
3. Legal and Regulatory Challenges in AI
A. Intellectual property and patenting of AI technology
One of the legal and regulatory challenges related to AI is the issue of intellectual property and patenting of AI technology. As AI systems become more advanced, they are generating intellectual property that may be subject to patent protection. However, there is a debate about whether AI systems can be considered inventors or whether the humans who created the AI should be listed as the inventors. This has implications for patent law and the ownership of AI-generated inventions.
B. Liability and responsibility for AI decisions
Second legal and regulatory challenge related to AI is the issue of liability and responsibility for AI decisions. As AI systems become more autonomous, there is a risk that they may make decisions that have unintended consequences or that harm individuals or society. It is important to establish clear rules and guidelines for liability and responsibility for AI decisions to ensure that individuals and organizations are held accountable for the actions of their AI systems.
C. Regulation of autonomous systems and AI applications
Another legal and regulatory challenge related to AI is the regulation of autonomous systems and AI applications. As AI systems become more advanced and autonomous, there is a need for clear regulations and standards to ensure that these systems are safe, reliable, and transparent. This includes issues such as testing and certification of AI systems, as well as requirements for transparency and explainability in AI decision-making.
D. International cooperation and governance of AI
Finally, AI is a global technology that has the potential to impact countries and societies around the world. As such, there is a need for international cooperation and governance of AI to ensure that it is developed and used in a responsible and ethical manner. This includes issues such as setting global standards for AI development and use, as well as ensuring that there is equitable access to AI technologies and that they are not used to perpetuate existing power imbalances or inequalities.
4. Technical Challenges in AI
A. Reliability and interpretability of AI systems
One of the technical challenges related to AI is the issue of reliability and interpretability of AI systems. As AI systems become more complex and autonomous, there is a risk that they may make decisions that are unpredictable or difficult to interpret. This can be a challenge in fields such as healthcare or finance where decisions made by AI systems can have significant impacts on individuals or organizations. It is important to develop AI systems that are reliable and interpretable to ensure that they can be trusted and used effectively.
B. Robustness and security of AI systems
Next technical challenge related to AI is the issue of robustness and security of AI systems. AI systems are vulnerable to attacks and can be compromised if they are not designed with security in mind. This can result in significant consequences, including data breaches or the manipulation of AI decision-making. It is important to develop robust and secure AI systems to ensure that they can be used safely and effectively.
C. Explainability and transparency in AI decision-making
Subsequent technical challenge related to AI is the issue of explainability and transparency in AI decision-making. As AI systems become more autonomous and complex, it can be difficult to understand how they arrived at a particular decision. This can be a challenge in fields such as healthcare or finance where decisions made by AI systems can have significant impacts on individuals or organizations. It is important to develop AI systems that are explainable and transparent to ensure that they can be trusted and used effectively.
D. Advancements in AI and potential future challenges
Finally, AI is a rapidly evolving technology, and there are likely to be new technical challenges that arise as AI systems become more advanced. For example, as AI systems become more powerful, there is a risk that they may be used for malicious purposes, such as cyber attacks or disinformation campaigns. It is important to stay up-to-date with advancements in AI and to be prepared to address potential future challenges as they arise.
5. Case Studies and Examples
A. Real-world examples of AI’s impact on critical issues
One way to explore the impact of AI on critical issues is to examine real-world examples. For instance, AI-powered facial recognition technology has been criticized for perpetuating bias and discrimination against marginalized communities. In healthcare, AI systems have been used to predict patient outcomes and improve treatment, but there are concerns about the privacy and security of patient data. Another example is the use of AI in financial markets, which has the potential to disrupt traditional models of investing and trading.
B. Case studies on ethical and social implications of AI
Case studies can provide insight into the ethical and social implications of AI. For example, the case of Tay, Microsoft’s AI chatbot, provides a cautionary tale about the potential dangers of releasing an AI system into the wild without proper safeguards. The case of Amazon’s recruitment tool, which was found to be biased against women, highlights the importance of addressing bias and discrimination in AI algorithms.
C. Legal and regulatory challenges faced by AI companies and policymakers
Case studies can also highlight the legal and regulatory challenges faced by AI companies and policymakers. For example, the case of Waymo vs. Uber, in which Waymo accused Uber of stealing its self-driving car technology, illustrates the complexity of intellectual property and patent law in the context of AI. The case of the GDPR and its impact on AI companies operating in Europe highlights the challenges of regulating AI in a rapidly changing technological landscape.
D. Technical challenges and solutions in AI research and development
Finally, case studies can shed light on the technical challenges and solutions in AI research and development. For example, the case of AlphaGo, the AI system that defeated a world champion at the game of Go, demonstrates the power of deep learning and reinforcement learning in developing advanced AI systems. The case of OpenAI’s GPT-3 language model, which can generate coherent and convincing text, highlights the potential of AI for natural language processing and text generation.
6. Future Directions and Recommendations
A. Predictions for the future of AI and its impact on critical issues
As AI continues to evolve and become more advanced, it is likely to have a significant impact on critical issues such as healthcare, climate change, and economic inequality. For example, AI could be used to improve healthcare outcomes by providing personalized treatments and predicting disease outbreaks. AI could also be used to address climate change by optimizing energy use and reducing waste. However, there are also risks and challenges associated with AI, such as the potential for AI to perpetuate bias and discrimination or to be used for malicious purposes.
B. Recommendations for policymakers, researchers, and industry leaders
To ensure that AI is used safely and effectively, policymakers, researchers, and industry leaders must work together to develop ethical and responsible AI systems. This includes addressing issues such as bias and discrimination in AI algorithms, ensuring the privacy and security of data, and developing regulations to govern the use of AI. It is also important to invest in AI research and development to continue advancing the technology and to ensure that it is used for the public good.
C. Opportunities for collaboration and interdisciplinary research
AI is a complex and interdisciplinary field, and there are many opportunities for collaboration and interdisciplinary research. For example, researchers in computer science, psychology, and neuroscience can work together to develop AI systems that are more human-like in their decision-making. Collaboration between industry and academia can also help to ensure that AI systems are developed responsibly and with consideration for their social and ethical implications.
D. Conclusion and final thoughts
AI has the potential to revolutionize many aspects of society, but it is important to approach its development and use with caution and responsibility. By addressing the technical, ethical, and social challenges of AI, we can ensure that it is used for the public good and that its benefits are shared equitably. It is up to policymakers, researchers, and industry leaders to work together to develop AI systems that are ethical, responsible, and trustworthy.
7. Glossary of key terms and concepts
Here are some key terms and concepts related to AI that may be helpful to include in a glossary:
- Artificial Intelligence (AI) – The simulation of human intelligence in machines, including learning, reasoning, and perception.
- Machine Learning – A subfield of AI that allows machines to learn from data and improve over time without being explicitly programmed.
- Deep Learning – A type of machine learning that uses artificial neural networks to analyze and learn from data.
- Neural Networks – A type of machine learning algorithm that is modeled after the structure and function of the human brain.
- Natural Language Processing (NLP) – A subfield of AI that focuses on the interaction between computers and human language, including speech recognition and machine translation.
- Bias – The presence of unfairness or prejudice in data or algorithms, resulting in unequal treatment or outcomes for different groups.
- Ethics – A set of moral principles that guide human behavior and decision-making.
- Transparency – The ability to understand and explain how AI algorithms make decisions and operate.
- Privacy – The right to control access to one’s personal information and data.
- Regulation – The process of creating and enforcing rules and guidelines for the use of AI and other technologies.
- Autonomous Systems – Systems that can operate and make decisions without human intervention.
- Responsible AI – The development and use of AI systems that are ethical, transparent, and accountable.
- Robotics – A branch of engineering and AI that deals with the design, construction, and operation of robots.
- Algorithm – A set of instructions or rules that a computer or machine follows to perform a specific task.
- Big Data – Extremely large datasets that can be analyzed to reveal patterns, trends, and insights.
- Data Mining – The process of extracting patterns and knowledge from large datasets using machine learning and statistical techniques.
- Computer Vision – A subfield of AI that focuses on enabling machines to interpret and understand visual information from the world around them.
- Chatbot – An AI-powered program or system that simulates human conversation through text or voice interactions.
- Explainable AI – The ability of AI systems to provide clear and understandable explanations of how they arrived at a decision or recommendation.
- Adversarial Examples – Inputs to AI systems that are specifically designed to cause them to make incorrect or misleading predictions or decisions.
- Supervised Learning – A type of machine learning where the AI system is trained using labeled data, which is data that has already been categorized or classified by humans.
- Unsupervised Learning – A type of machine learning where the AI system is trained using unlabeled data, and the system must discover patterns and relationships on its own.
- Reinforcement Learning – A type of machine learning where the AI system learns through trial and error, receiving feedback in the form of rewards or punishments.
- Human-in-the-Loop – A type of AI system where human input is integrated into the learning and decision-making process to improve accuracy and reduce bias.
- Singularity – A hypothetical point in the future when AI becomes capable of recursive self-improvement, leading to an exponential increase in intelligence that surpasses human intelligence.
References:
- https://ai100.stanford.edu/2016-report
- “Artificial Intelligence: A Guide to the Technology and Its Future” book by Jerry Kaplan, which provides an overview of AI, its history, and its potential impacts on society.
- “AI Now Report” by the AI Now Institute, which identifies and addresses issues related to the social implications of AI, including bias, accountability, and transparency.
Related Topics:
https://amateurs.co.in/ai-and-job-automation-its-impacts/
https://amateurs.co.in/ethical-values-in-the-synergy-of-ai-and-blockchain/
https://amateurs.co.in/how-ai-is-contributing-to-water-scarcity/
https://amateurs.co.in/ai-vs-ml-vs-iot-vs-robotics-vs-nlp-vs-deep-learning/
https://amateurs.co.in/what-is-natural-language-processing-nlp/
https://amateurs.co.in/what-is-chatgpt-and-how-does-it-work/
[…] https://amateurs.co.in/critical-issues-in-artificial-intelligence/ […]