Quote:
“Language is the road map of a culture. It tells you where its people come from and where they are going.”
This quote was said by the American writer and anthropologist Rita Mae Brown. While not specifically about NLP, it highlights the importance of language as a key component of culture and communication, which NLP seeks to understand and utilize in the development of AI technologies.
Table of Contents
Introduction:
Customer service has always been an essential part of any business, but it can also be time-consuming and costly. However, recent advances in natural language processing (NLP) are making it easier and more cost-effective than ever to provide high-quality customer service. In this blog post, we’ll explore how NLP is revolutionizing the world of customer service and what it means for businesses and their customers.
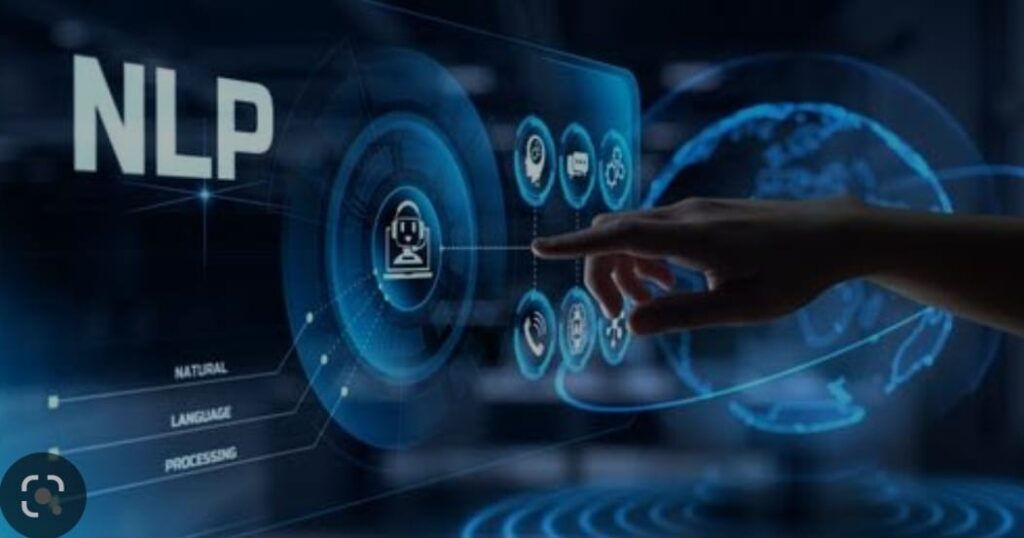
Section 1: Understanding Natural Language Processing
In this section, we’ll provide an overview of what NLP is and how it works. We’ll explain the difference between rule-based and machine learning-based approaches to NLP and discuss the challenges and opportunities of each approach.
Natural Language Processing (NLP) is a branch of artificial intelligence that focuses on the interaction between humans and computers using natural language. NLP systems enable computers to understand, interpret, and generate human language, which can be a valuable tool for businesses, researchers, and individuals.
There are two main approaches to NLP: rule-based and machine learning-based. Rule-based NLP relies on a set of predefined rules that the system uses to analyze text and make decisions about how to respond. These rules are typically created by linguistic experts and can be useful for simple tasks, such as identifying parts of speech or basic sentiment analysis. However, rule-based systems are often limited in their ability to understand and respond to complex language.
On the other hand, machine learning-based NLP uses algorithms and statistical models to analyze language and identify patterns. These models are trained on large amounts of data and can be more accurate and flexible than rule-based systems. Machine learning-based NLP can also be used for more complex tasks, such as language translation, chatbot interactions, and sentiment analysis.
While machine learning-based NLP has many advantages, there are also challenges associated with this approach. One major challenge is the need for large amounts of high-quality training data to build accurate models. Additionally, machine learning-based NLP systems can be susceptible to bias, as they may learn from biased data sets or use biased algorithms.
Some important point about NLP:-
Here are some important points to know about NLP:
- NLP is a subfield of artificial intelligence that focuses on enabling computers to understand, interpret, and generate human language.
- NLP technology is used in a wide range of applications, including speech recognition, machine translation, sentiment analysis, and chatbots.
- There are two main approaches to NLP: rule-based and machine learning-based. Rule-based systems use handcrafted rules to analyze language, while machine learning-based systems use statistical models to learn from data.
- NLP faces several challenges, including ambiguity, context-dependency, and the need to understand idiomatic expressions and sarcasm.
- Recent advances in deep learning and neural networks have led to significant improvements in NLP performance, particularly in areas such as machine translation and natural language generation.
- NLP has the potential to revolutionize a wide range of industries, including healthcare, finance, and customer service, by enabling more efficient and personalized communication with customers and patients.
- As NLP technology continues to advance, ethical considerations around privacy, bias, and accountability will become increasingly important.
Overall, both rule-based and machine learning-based NLP approaches have their strengths and weaknesses, and the choice of which approach to use will depend on the specific application and use case. As NLP technology continues to advance, we can expect to see new and innovative approaches to language processing emerge.
Section 2: How NLP is Improving Customer Service
In this section, we’ll delve into the ways in which NLP is transforming the customer service experience. We’ll explore how NLP-powered chatbots can handle customer inquiries and support tickets, and how they can be customized to understand the unique needs and preferences of each customer.
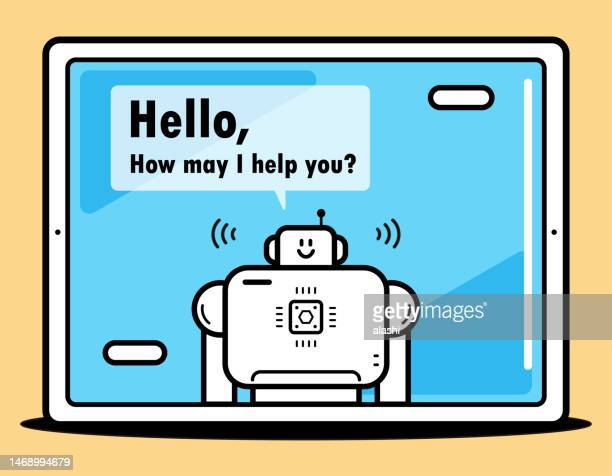
NLP is transforming the customer service experience in many ways, and one of the most significant is through the use of NLP-powered chatbots. These chatbots can handle customer inquiries and support tickets in a fast, efficient, and personalized manner, improving customer satisfaction and reducing costs for businesses.
NLP-powered chatbots use natural language processing to understand and interpret customer inquiries and respond appropriately. They can handle a wide variety of inquiries, from simple questions about product features to more complex technical support issues. This frees up human customer service agents to focus on more challenging inquiries that require human intervention, while also ensuring that customers receive prompt and accurate responses to their inquiries.
In addition, NLP-powered chatbots can be customized to understand the unique needs and preferences of each customer. By analyzing previous customer interactions, chatbots can learn about each customer’s interests, preferences, and behavior patterns. This information can be used to personalize the customer service experience, tailoring responses to each customer’s specific needs and preferences.
Section 3: Real-World Examples of NLP in Action
In this section, we’ll showcase some real-world examples of companies that are using NLP to provide better customer service. We’ll highlight companies from a variety of industries and discuss the specific NLP technologies they are using to improve their customer service offerings.
There are many real-world examples of companies that are using NLP to provide better customer service. Here are a few:
- American Express: American Express uses NLP to power its chatbot, which can answer customer inquiries, provide account information, and even help customers book travel. The chatbot uses machine learning algorithms to improve its understanding of customer inquiries over time, resulting in faster and more accurate responses.
- H&M: H&M uses NLP to power its voice-activated fashion assistant, which allows customers to search for items and get fashion advice using natural language. The system uses deep learning algorithms to understand customer inquiries and provide personalized recommendations based on their preferences.
- Pizza Hut: Pizza Hut uses NLP to power its chatbot, which can handle customer inquiries, take orders, and even provide recommendations for menu items. The chatbot uses natural language understanding and machine learning algorithms to improve its responses over time and provide a more personalized customer experience.
- KLM Royal Dutch Airlines: KLM uses NLP to power its social media customer service, which allows customers to ask questions and receive answers in multiple languages. The system uses machine learning algorithms to analyze customer inquiries and route them to the appropriate customer service agent, resulting in faster response times and higher customer satisfaction.
- MD India: MD India uses NLP to power its Chatbot Pepper, which can handle customer inquiries, provide account information, and even assist with Claim Status. The virtual assistant uses machine learning algorithms to improve its understanding of customer inquiries and provide more personalized responses over time. Follow link to know more http://mdichatbot.mdindia.com/mdichat/mdichat.aspx
Overall, these examples demonstrate how NLP can be used to improve the customer service experience across a variety of industries. By using natural language understanding and machine learning algorithms, businesses can provide faster, more accurate, and more personalized responses to customer inquiries, resulting in higher customer satisfaction and loyalty.
Section 4: Future Trends in NLP and Customer Service
In this section, we’ll look to the future and explore the trends that are likely to shape the intersection of NLP and customer service. We’ll discuss the emergence of voice-based interfaces and the potential for NLP to provide personalized, proactive customer service.
The future of NLP and customer service is exciting, with many emerging trends that have the potential to transform the way businesses interact with their customers. Here are a few key trends to watch for:
- Voice-based interfaces: With the rise of smart speakers and voice assistants, voice-based interfaces are becoming an increasingly popular way for customers to interact with businesses. NLP technology is essential for enabling these voice-based interfaces to understand and interpret natural language, and we can expect to see more businesses leveraging this technology to provide voice-activated customer service in the future.
- Proactive customer service: NLP technology has the potential to enable businesses to provide personalized, proactive customer service by analyzing customer data and anticipating their needs. For example, NLP algorithms could analyze a customer’s past purchases and preferences to make personalized recommendations or alert the customer when a relevant product or service becomes available.
- Emotional intelligence: As NLP technology becomes more advanced, we may see the emergence of chatbots and virtual assistants that can not only understand the literal meaning of language but also interpret emotions and respond appropriately. This could enable businesses to provide more empathetic and compassionate customer service, improving customer satisfaction and loyalty.
- Multilingual support: As businesses continue to expand globally, the ability to provide multilingual customer support will become increasingly important. NLP technology can enable businesses to provide support in multiple languages, allowing them to better serve customers around the world.
Overall, the future of NLP and customer service is full of potential. By leveraging the latest advances in NLP technology, businesses can provide more personalized, proactive, and empathetic customer service, improving customer satisfaction and driving business success.
Conclusion:
In conclusion, NLP is transforming the customer service landscape in exciting ways. By leveraging the power of machine learning and natural language processing, businesses can provide better, more efficient customer service while also reducing costs and improving customer satisfaction.
NLP-powered chatbots and virtual assistants have the ability to handle customer inquiries, provide personalized recommendations, and even assist with financial planning. Furthermore, with the emergence of voice-based interfaces and proactive customer service, businesses can offer even more tailored and effective solutions to their customers. As NLP technology continues to advance, we can expect to see even more innovative applications in customer service, such as emotional intelligence and multilingual support. Ultimately, NLP is a powerful tool that businesses can use to improve the customer experience and gain a competitive advantage in today’s digital landscape.
Glossary on NLP
- Natural Language Processing (NLP): A subfield of artificial intelligence that focuses on enabling computers to understand, interpret, and generate human language.
- Machine Learning: A subset of AI that enables computers to learn from data without being explicitly programmed.
- Rule-Based Systems: NLP systems that use handcrafted rules to analyze language.
- Corpus: A large and structured set of texts used to develop and train NLP models.
- Part-of-Speech Tagging: A process in NLP that involves assigning grammatical labels to each word in a sentence, such as noun, verb, or adjective.
- Sentiment Analysis: An NLP technique that involves identifying the sentiment expressed in a piece of text, such as positive, negative, or neutral.
- Named Entity Recognition: An NLP technique that involves identifying and extracting named entities, such as people, organizations, and locations, from text.
- Stemming: An NLP technique that involves reducing words to their root form to improve text analysis and retrieval.
- Stop Words: Common words, such as “the” and “and,” that are removed from text during NLP analysis to improve efficiency and accuracy.
- Vectorization: The process of converting text data into numerical vectors for machine learning analysis.
- Deep Learning: A subset of machine learning that uses artificial neural networks to model complex relationships in data.
- Natural Language Understanding (NLU): An NLP task that involves analyzing text to extract meaning and intent.
- Natural Language Generation (NLG): An NLP task that involves generating human-like text based on structured data.
- Chatbot: An AI-powered conversational agent that can communicate with humans using natural language.
- Semantic Analysis: An NLP technique that involves understanding the meaning of words and phrases in context.
- Dependency Parsing: An NLP task that involves analyzing the grammatical relationships between words in a sentence.
- Language Model: An NLP model that predicts the probability of a sequence of words in a sentence.
- Named Entity Disambiguation: An NLP technique that involves identifying the correct entity when there are multiple entities with the same name.
- Topic Modeling: An NLP technique that involves identifying the topics present in a set of documents.
- Ontology: A formal representation of knowledge that includes a set of concepts and the relationships between them, used in NLP to enable computers to understand the meaning of language.
Reference:
Related Topics
https://amateurs.co.in/ai-and-job-automation-its-impacts/
https://amateurs.co.in/ethical-values-in-the-synergy-of-ai-and-blockchain/
https://amateurs.co.in/how-ai-is-contributing-to-water-scarcity/
https://amateurs.co.in/ai-vs-ml-vs-iot-vs-robotics-vs-nlp-vs-deep-learning/
https://amateurs.co.in/what-is-natural-language-processing-nlp/
https://amateurs.co.in/what-is-chatgpt-and-how-does-it-work/