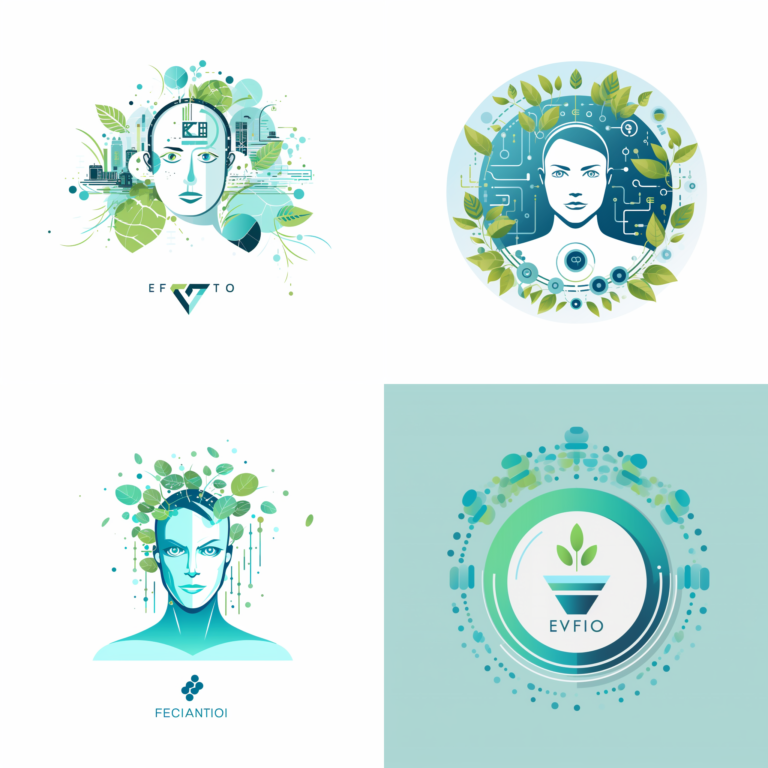
the Ethics of using AI in mental health diagnosis is of utmost importance. Privacy and data security must be upheld, while bias and fairness need to be actively addressed. Respecting informed consent and patient autonomy ensures their involvement in decision-making. Striking the right balance between AI and human involvement is crucial for responsible and ethical implementation.
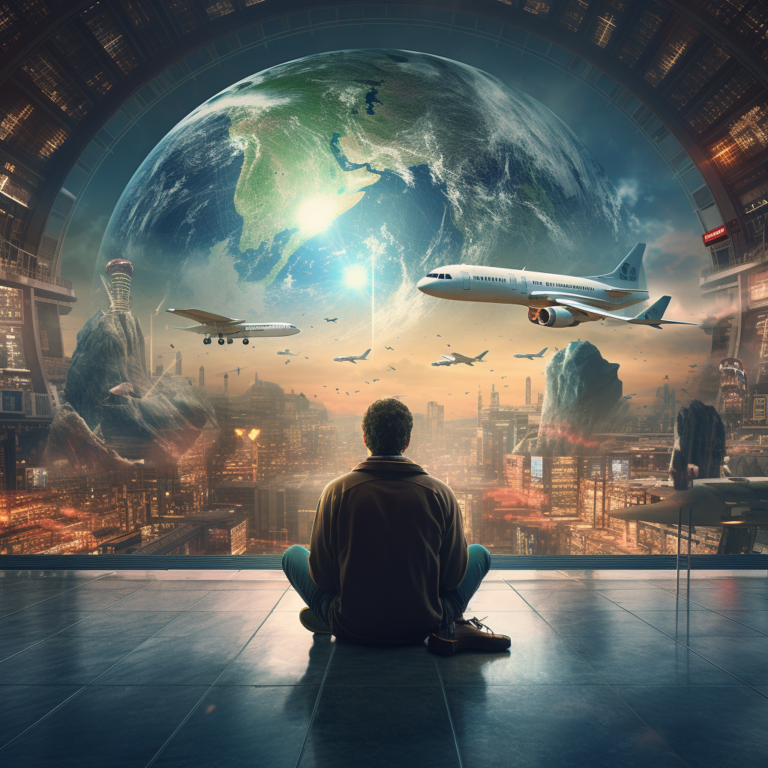
Human-AI collaboration is a groundbreaking synergy that unites human intelligence and the computational power of AI systems. It is a dynamic partnership where human expertise, intuition, and creativity intertwine with the speed, efficiency, and analytical capabilities of AI. Together, humans and AI systems are transforming industries, redefining problem-solving approaches, and reshaping the boundaries of what we thought was achievable.
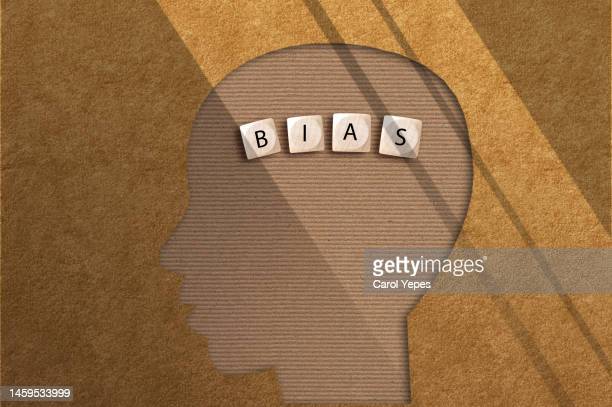
Bias and fairness in AI are critical issues that demand attention. AI algorithms can inadvertently encode biases, leading to discriminatory outcomes. Ensuring fairness in AI decision-making is essential to mitigate harm and uphold ethical standards. Transparency, diverse data, and algorithmic fairness techniques play crucial roles in addressing biases and promoting a more inclusive and equitable AI ecosystem.
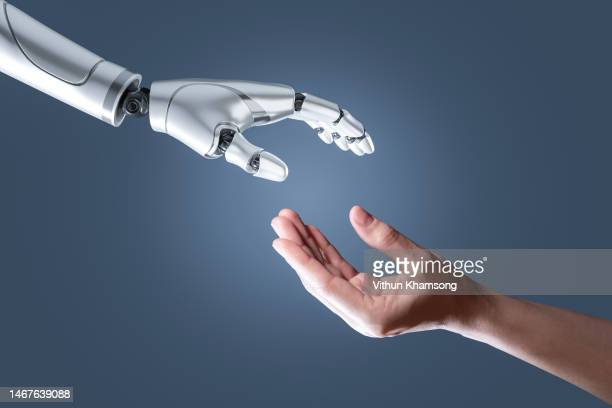
Explainable AI (XAI) is a field of research that seeks to make AI models more transparent and interpretable. By improving the explainability of AI, XAI aims to enhance trust, accountability, and ethical considerations in AI development and deployment. XAI techniques can be applied to a wide range of AI models and applications, including natural language processing, image recognition, and predictive modeling.