“The ethical integration of AI in mental health diagnosis ensures that innovation is driven by compassion, privacy, and human connection, empowering us to harness technology responsibly for the well-being of individuals seeking mental health support.”
Table of Contents
1.Introduction: Unveiling the Role of AI in Mental Health Diagnosis
In recent years, the field of mental health has witnessed significant advancements in technology, particularly in the realm of artificial intelligence (AI). AI refers to the development of computer systems capable of performing tasks that typically require human intelligence, such as learning, reasoning, and problem-solving. When applied to mental health diagnosis, AI has the potential to revolutionize the way we understand, assess, and treat various mental health conditions.
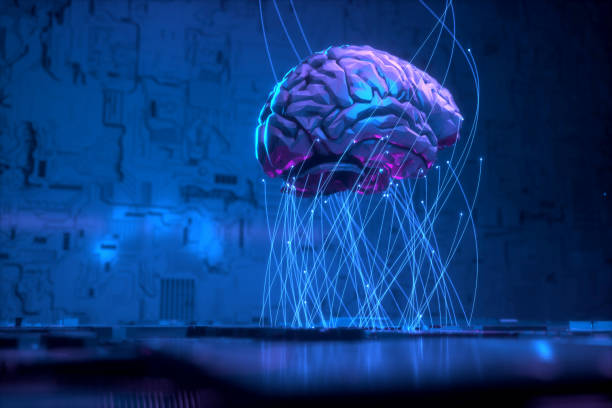
The use of AI in mental health diagnosis involves the analysis of vast amounts of data, including patient information, symptom profiles, and treatment outcomes. By employing sophisticated algorithms, AI can detect patterns, predict outcomes, and provide valuable insights to healthcare professionals. This technology holds promise for improving the accuracy, efficiency, and accessibility of mental health diagnosis.
2.Understanding the Potential of AI in Mental Health Diagnosis
2.1 Advantages of AI in Mental Health Diagnosis
The integration of AI in mental health diagnosis offers numerous advantages that can revolutionize the field. Let’s explore some of these advantages:
a) Enhanced Data Analysis: AI can process vast amounts of patient data, including medical records, diagnostic assessments, and even data from wearable devices. This enables AI algorithms to detect patterns and relationships that may not be apparent to human clinicians. For example, AI can identify subtle changes in speech patterns or facial expressions that may indicate the presence of certain mental health conditions.
b) Early Detection and Intervention: AI algorithms can help in the early detection of mental health disorders by analyzing a wide range of data points. This allows for timely intervention and treatment, which can significantly improve patient outcomes. For instance, AI can analyze social media posts and detect warning signs of depression or anxiety, enabling healthcare professionals to intervene and offer support.
c) Personalized Treatment Plans: AI can assist in creating personalized treatment plans based on individual patient data. By considering various factors such as symptoms, medical history, and genetic information, AI algorithms can generate tailored recommendations for treatment approaches and medications. This ensures that patients receive the most effective and suitable interventions for their specific needs.
d) Improved Efficiency and Accuracy: AI technology can help streamline the diagnostic process, reducing the time and resources required for accurate assessments. AI algorithms can rapidly analyze large datasets, compare symptoms against established diagnostic criteria, and generate reliable results. This efficiency can lead to faster diagnoses and more timely interventions.
2.2 Limitations and Challenges
While AI holds immense promise, there are important limitations and challenges that must be addressed:
a) Reliability and Interpretability: The reliability and interpretability of AI algorithms can be a concern. It can be challenging to understand the decision-making process of complex AI models, making it difficult to trust their results. Additionally, AI algorithms can be sensitive to biases present in the training data, leading to potentially skewed or inaccurate predictions. Efforts must be made to ensure transparency and accountability in AI systems.
b) Ethical and Privacy Concerns: The use of AI in mental health diagnosis raises ethical considerations regarding patient privacy and data security. Confidential patient information must be handled with utmost care to protect individual privacy. Clear guidelines and protocols need to be in place to address these concerns and safeguard patient data.
c) Human Connection and Emotional Understanding: AI technologies lack the ability to fully understand and empathize with human emotions and experiences. While AI can provide valuable insights, it cannot replace the human connection and therapeutic alliance that is crucial in mental health treatment. Maintaining a balance between AI-assisted diagnosis and human interaction is essential to ensure comprehensive and compassionate care.
d) Accessibility and Equity: The widespread adoption of AI in mental health diagnosis should consider issues of accessibility and equity. AI technologies should be designed with considerations for diverse populations and should not perpetuate existing disparities in healthcare. Efforts must be made to ensure that AI tools are accessible to all individuals, regardless of socioeconomic status or geographic location.
By understanding the potential advantages of AI in mental health diagnosis and addressing the associated limitations and challenges, we can strive for responsible and ethical integration of this technology into mental healthcare systems.
3.Ethical Considerations in AI-assisted Mental Health Diagnosis
3.1 Privacy and Data Security
The use of AI in mental health diagnosis involves the collection and analysis of sensitive patient data. Privacy and data security are paramount in maintaining patient trust and ensuring ethical practices. Here are some key considerations:
a) Data Protection: Adequate measures should be in place to protect patient data from unauthorized access, breaches, or misuse. Robust encryption, secure storage, and stringent access controls are essential to safeguard patient privacy.
b) Anonymization and De-identification: Patient data used in AI algorithms should be appropriately anonymized and de-identified to ensure that individuals cannot be personally identified from the data. This protects patient confidentiality while allowing for valuable analysis and research.
c) Consent and Transparency: Patients should be informed about the use of AI in their diagnosis and have the right to provide informed consent. Healthcare providers should clearly communicate how AI technology will be utilized, the potential benefits and risks, and how patient data will be protected. Transparency about data handling practices builds trust between patients and healthcare professionals.
3.2 Bias and Fairness
AI algorithms are trained on data, and if the training data contains biases, those biases can be perpetuated in the AI system. Addressing bias and ensuring fairness in AI-assisted mental health diagnosis is crucial. Considerations include:
a) Diverse and Representative Data: AI algorithms should be trained on diverse and representative datasets that accurately reflect the population. Including data from different demographics and cultural backgrounds can help mitigate bias and ensure fairness in diagnosis and treatment recommendations.
b) Regular Monitoring and Evaluation: Continuous monitoring and evaluation of AI systems are necessary to identify and mitigate bias. Regular audits and assessments should be conducted to ensure that the algorithms are providing fair and equitable results across different populations.
c) Explainability and Accountability: AI algorithms should be transparent and explainable, allowing clinicians and patients to understand how decisions are made. Clear explanations help identify potential biases and enable accountability for any errors or unintended consequences that may arise.
3.3 Informed Consent and Autonomy
Respecting patient autonomy and ensuring informed consent are vital ethical considerations in AI-assisted mental health diagnosis:
a) Informed Decision-making: Patients should be provided with clear and understandable information about the use of AI in their diagnosis and treatment. This includes explanations of how AI technology works, its limitations, and potential implications. Informed consent empowers patients to make choices about their own healthcare.
b) Human Oversight and Collaboration: While AI can provide valuable insights, it should never replace human judgment and decision-making. Healthcare professionals should maintain a central role in diagnosis and treatment planning, incorporating AI as a supportive tool rather than a replacement. Collaborative decision-making ensures that patient preferences and values are considered.
c) Regular Evaluation and Improvement: Continuous evaluation of AI systems is necessary to assess their accuracy, effectiveness, and impact on patient well-being. Patient feedback and experiences should be actively sought and incorporated to enhance the ethical use of AI in mental health diagnosis.
By addressing privacy concerns, mitigating bias, and upholding informed consent and autonomy, the ethical integration of AI in mental health diagnosis can be promoted, leading to improved patient outcomes and trust in the healthcare system.
4.The Impact of AI on the Therapist-Patient Relationship
4.1 Enhancing Diagnostic Accuracy and Treatment Planning
AI has the potential to enhance diagnostic accuracy and treatment planning in mental health by providing healthcare professionals with valuable insights and support. Here’s how AI can contribute:
a) Objective Analysis: AI algorithms can analyze large amounts of patient data, including symptoms, medical history, and treatment outcomes. By considering a wide range of factors, AI can assist in identifying patterns and correlations that may not be immediately apparent to human clinicians. This objective analysis can lead to more accurate diagnoses and tailored treatment plans.
b) Evidence-Based Recommendations: AI can leverage vast databases of clinical research and best practices to provide evidence-based recommendations for treatment approaches. This can help healthcare professionals stay up-to-date with the latest research and ensure that treatment plans are aligned with current evidence.
c) Efficiency and Time-saving: AI can automate certain tasks, such as data analysis and documentation, freeing up healthcare professionals’ time to focus on direct patient care. This increased efficiency allows therapists to spend more quality time with their patients, improving overall treatment outcomes.
4.2 Risks of Overreliance on AI
While AI can be a valuable tool, overreliance on AI in mental health diagnosis poses certain risks that must be considered:
a) Diagnostic Inaccuracy: AI systems are not infallible and can make errors or produce false positives/negatives. Relying solely on AI without human oversight and critical analysis may lead to inaccurate diagnoses or treatment decisions. It is crucial for healthcare professionals to exercise clinical judgment and validate AI-generated recommendations.
b) Lack of Contextual Understanding: AI algorithms may lack the ability to fully comprehend the nuances of a patient’s unique circumstances and subjective experiences. Human therapists bring a holistic understanding of the patient’s background, cultural factors, and individual preferences. Maintaining a balance between AI-driven insights and human expertise is essential to avoid overlooking important contextual information.
c) Impact on Therapeutic Alliance: The therapeutic alliance, built on trust, empathy, and human connection, is a fundamental aspect of mental health treatment. Overreliance on AI may undermine the patient’s perception of being understood and heard. Maintaining a human-centered approach and incorporating AI as a supplementary tool can help preserve and strengthen the therapist-patient relationship.
4.3 Maintaining Human Connection and Empathy
While AI can provide valuable support, maintaining human connection and empathy in mental health diagnosis is crucial. Here’s why:
a) Emotionally Responsive Care: AI lacks the ability to empathize and understand the complex emotions and nuances of human experiences. Human therapists can provide emotional support, validate feelings, and respond to the unique needs of each patient. This personalized care fosters trust and emotional healing.
b) Communication and Rapport: Building rapport and trust with a therapist often relies on effective communication, active listening, and non-verbal cues. These elements of human interaction are essential for establishing a therapeutic alliance. Human therapists can adapt their approach based on patient responses, creating a safe and supportive environment.
c) Ethical Considerations: Ethical principles in mental health emphasize the importance of respect, autonomy, and empathy. Human therapists are uniquely equipped to navigate ethical dilemmas, complex emotions, and sensitive conversations with patients. They can consider individual values, cultural backgrounds, and personal circumstances in decision-making processes.
Striking a balance between AI-driven insights and human connection ensures a comprehensive and patient-centered approach to mental health diagnosis and treatment. By leveraging the advantages of AI while preserving the vital role of human therapists, we can maximize the benefits of both for the well-being of patients.
5.Addressing Ethical Concerns: Guidelines and Regulations
5.1 Establishing Ethical Frameworks for AI Integration
To address the ethical concerns surrounding AI in mental health diagnosis, it is essential to establish clear ethical frameworks that guide its integration. Here are some key considerations:
a) Ethical Guidelines: Professional organizations, regulatory bodies, and policymakers should collaborate to develop comprehensive ethical guidelines specifically tailored to AI-assisted mental health diagnosis. These guidelines should outline principles, best practices, and standards to ensure responsible and ethical use of AI technology.
b) Multidisciplinary Collaboration: Ethical frameworks should involve input from diverse stakeholders, including mental health professionals, AI experts, ethicists, and patient advocacy groups. Incorporating multiple perspectives ensures a comprehensive and balanced approach to addressing ethical considerations.
c) Continuous Review and Adaptation: Ethical frameworks should be regularly reviewed and updated to keep pace with evolving technology and emerging ethical challenges. Ongoing collaboration and feedback from stakeholders are crucial to ensure that ethical guidelines remain relevant and effective.
5.2 Ensuring Transparency and Explainability
Transparency and explainability are vital in fostering trust and addressing ethical concerns related to AI-assisted mental health diagnosis. Consider the following:
a) Clear Communication: Healthcare professionals should clearly communicate to patients how AI technology is being used in their diagnosis, treatment planning, and ongoing care. Patients should understand the limitations, potential biases, and risks associated with AI, as well as the benefits it can provide.
b) Explainable AI: Efforts should be made to develop AI algorithms that are transparent and explainable. Researchers and developers should work towards creating models that can provide clear explanations for their decisions and predictions, allowing clinicians and patients to understand the reasoning behind AI-generated recommendations.
c) Algorithmic Audits: Regular audits and assessments of AI algorithms should be conducted to identify and address potential biases, errors, or unintended consequences. These audits help ensure that AI systems operate in a fair, accurate, and transparent manner.
5.3 Strengthening Legal and Regulatory Measures
To promote ethical AI integration in mental health diagnosis, legal and regulatory measures play a vital role. Consider the following steps:
a) Data Privacy Regulations: Governments should enact and enforce robust data privacy regulations that safeguard patient information in AI systems. These regulations should outline strict requirements for data handling, storage, and consent to protect patient privacy.
b) Bias and Fairness Assessments: Regulatory bodies can establish guidelines for assessing and mitigating bias in AI algorithms used in mental health diagnosis. Regular audits and assessments should be conducted to evaluate the fairness and equity of AI systems across different populations.
c) Compliance and Accountability: Legal frameworks should outline the responsibilities and obligations of healthcare providers, AI developers, and stakeholders involved in AI-assisted mental health diagnosis. Clear lines of accountability ensure that ethical standards are upheld, and appropriate actions are taken in cases of negligence or ethical violations.
By establishing ethical frameworks, ensuring transparency and explainability, and strengthening legal and regulatory measures, we can foster responsible and ethical integration of AI in mental health diagnosis. These measures protect patient rights, promote fairness, and build public trust in the use of AI technologies for mental healthcare.
6.Balancing Innovation and Patient Well-being: A Holistic Approach
6.1 Integrating AI as a Complementary Tool
To strike a balance between innovation and patient well-being, AI should be integrated as a complementary tool in mental health diagnosis. Here’s how this can be achieved:
a) Augmenting Clinical Expertise: AI should be seen as a supportive tool that augments the clinical expertise of healthcare professionals. It can assist in data analysis, pattern recognition, and evidence-based recommendations, providing valuable insights that complement the knowledge and experience of therapists.
b) Human Oversight and Decision-making: While AI algorithms can provide insights, the final decisions regarding diagnosis and treatment should involve the expertise and judgment of healthcare professionals. Human oversight ensures that clinical judgment, intuition, and contextual understanding are considered alongside AI-generated information.
c) Continual Learning and Adaptation: AI systems should be designed to learn and adapt over time based on feedback from healthcare professionals and patient outcomes. Continuous improvement and refinement of AI algorithms help ensure their relevance, accuracy, and effectiveness in supporting mental health diagnosis.
6.2 Promoting Collaboration between AI and Healthcare Professionals
Collaboration between AI technology and healthcare professionals is crucial to maximize the benefits and address ethical considerations. Consider the following:
a) Interdisciplinary Collaboration: Collaboration between mental health professionals, AI researchers, data scientists, and technologists is essential for effective integration. This interdisciplinary approach ensures that the strengths and limitations of AI are understood and applied in a manner that aligns with clinical best practices.
b) Training and Education: Healthcare professionals should receive training and education on AI technology, its applications, and its ethical implications. This equips them with the knowledge and skills needed to effectively collaborate with AI systems and interpret their outputs in a clinical context.
c) Feedback Loop: Open channels of communication should be established between healthcare professionals and AI developers. Feedback from clinicians regarding the usability, accuracy, and practicality of AI tools is valuable in driving improvements and refining algorithms to better meet the needs of patients and healthcare providers.
6.3 Prioritizing Patient Safety and Mental Health Outcomes
Innovation should always prioritize patient safety and mental health outcomes. Here are key considerations:
a) Risk Management: Thorough risk assessments should be conducted to identify potential risks associated with AI-assisted mental health diagnosis. Mitigation strategies should be implemented to minimize risks and ensure patient safety. Regular monitoring and reporting of adverse events or errors should be encouraged to continuously improve AI systems.
b) Outcome Evaluation: Continuous evaluation of AI-assisted mental health diagnosis is essential to assess its impact on patient outcomes. Monitoring treatment efficacy, patient satisfaction, and long-term well-being helps identify areas for improvement and ensures that AI technologies are contributing positively to mental health care.
c) Ethical Review Boards: Institutional ethical review boards should play a role in overseeing the integration of AI in mental health diagnosis. They can provide guidance, review research protocols, and evaluate the ethical implications of using AI technology, particularly in research studies involving human subjects.
By integrating AI as a complementary tool, promoting collaboration between AI and healthcare professionals, and prioritizing patient safety and mental health outcomes, a holistic approach can be achieved. This approach harnesses the potential of AI while upholding ethical standards and ensuring the well-being of individuals seeking mental health support.
7.Conclusion: Embracing Ethical AI in Mental Health Diagnosis
The use of AI in mental health diagnosis holds great promise for improving patient outcomes and enhancing the efficiency of healthcare delivery. However, it is essential to approach its integration with a strong focus on ethics, transparency, and patient well-being. By addressing ethical considerations and implementing responsible practices, we can ensure that AI becomes a valuable tool in mental healthcare.
Ultimately, embracing ethical AI in mental health diagnosis requires a holistic approach that balances innovation and patient well-being. By integrating AI as a complementary tool, promoting collaboration, and prioritizing patient safety and mental health outcomes, we can harness the potential of AI while upholding ethical standards and improving the lives of individuals seeking mental health support.
As technology continues to advance, it is vital for healthcare professionals, researchers, policymakers, and society as a whole to work together to shape the ethical landscape of AI in mental health diagnosis. By embracing ethical AI practices, we can navigate the complexities of this evolving field and ensure that AI remains a valuable and responsible asset in improving mental health care for all.
Glossary: The Ethics of Using AI in Mental Health Diagnosis
- AI (Artificial Intelligence): The field of computer science that focuses on developing intelligent machines capable of performing tasks that typically require human intelligence. In the context of mental health diagnosis, AI refers to the use of algorithms and machine learning techniques to analyze patient data and provide insights to assist in diagnosis and treatment planning.
- Ethics: The branch of philosophy that deals with moral principles and values, guiding human behavior and decision-making. In the context of AI in mental health diagnosis, ethics refers to the principles, guidelines, and considerations surrounding the responsible and ethical use of AI technology to ensure patient well-being, privacy, fairness, and autonomy.
- Privacy: The right of individuals to control access and use of their personal information. In AI-assisted mental health diagnosis, privacy refers to safeguarding patient data and ensuring secure storage, transmission, and handling of sensitive information to protect patient confidentiality and maintain trust.
- Data Security: Measures taken to protect data from unauthorized access, use, disclosure, or destruction. Data security in the context of AI in mental health diagnosis involves implementing robust security protocols, encryption, access controls, and ensuring compliance with data protection regulations to prevent data breaches and protect patient privacy.
- Bias: Systematic errors or prejudices that influence the outcome of an algorithm or decision-making process. In AI-assisted mental health diagnosis, bias refers to the potential for algorithms to produce unfair or discriminatory results based on factors such as race, gender, or socioeconomic status. Addressing and mitigating bias is essential to ensure equitable and unbiased diagnosis and treatment recommendations.
- Fairness: The principle of treating individuals equitably and without bias. Fairness in AI-assisted mental health diagnosis involves ensuring that AI algorithms do not disproportionately favor or discriminate against specific groups, providing equal opportunities and access to accurate diagnosis and appropriate treatment recommendations for all patients.
- Informed Consent: The voluntary and informed agreement of an individual to participate in a medical or research procedure after receiving complete information about the procedure, its risks, benefits, and alternatives. In the context of AI in mental health diagnosis, obtaining informed consent from patients is crucial to respect their autonomy and ensure they understand the use of AI technology in their diagnosis and treatment.
- Autonomy: The principle of respecting an individual’s right to make decisions and have control over their own life and healthcare. Autonomy in AI-assisted mental health diagnosis means involving patients in the decision-making process, ensuring their preferences and values are considered, and allowing them to have the final say in their diagnosis and treatment options.
- Therapist-Patient Relationship: The professional relationship between a therapist or mental health professional and their patient. In the context of AI in mental health diagnosis, the therapist-patient relationship refers to the importance of maintaining human connection, empathy, and trust during the integration of AI technology. It emphasizes the role of therapists in providing emotional support, personalized care, and the unique understanding of each patient’s context.
- Diagnostic Accuracy: The degree to which a diagnosis correctly identifies a patient’s mental health condition. AI-assisted mental health diagnosis aims to enhance diagnostic accuracy by analyzing large amounts of data, identifying patterns, and providing clinicians with objective insights to improve the accuracy and precision of diagnosis.
- Treatment Planning: The process of developing a tailored treatment plan based on a patient’s diagnosed mental health condition. AI in mental health diagnosis can assist in treatment planning by providing evidence-based recommendations, considering the patient’s unique characteristics, treatment outcomes, and current research, to optimize treatment approaches and improve patient outcomes.
- Overreliance on AI: The excessive dependence on AI technology without appropriate human oversight and critical analysis. Overreliance on AI in mental health diagnosis can lead to potential risks such as diagnostic inaccuracy, loss of human connection, and the disregard of important contextual factors. Striking a balance between AI and human expertise is crucial to avoid the pitfalls of overreliance and ensure responsible and effective use of AI technology.
- Human Connection: The emotional bond and interpersonal relationship between a patient and a healthcare professional. The ethical integration of AI in mental health diagnosis emphasizes the importance of maintaining human connection, empathy, and therapeutic rapport, even in the presence of AI technology. This ensures that patients receive holistic care that addresses their emotional needs, beyond the technical aspects of diagnosis and treatment.
- Empathy: The ability to understand and share the feelings, experiences, and perspectives of another person. Empathy is a vital component of the therapist-patient relationship and is essential in providing compassionate care. AI-assisted mental health diagnosis should complement and support the empathetic qualities of healthcare professionals, allowing them to deliver personalized and empathetic care to patients.
Related Articles
https://amateurs.co.in/the-emergent-properties-of-artificial-intelligence/
https://www.discovermagazine.com/technology/can-ai-read-your-mind
[…] https://amateurs.co.in/the-ethics-of-using-ai-in-mental-health-diagnosis/ […]